Deciphering the Future: Function of AlphaFold in Research
In the quest to decode the mysteries of aging, a groundbreaking artificial intelligence (AI) program called AlphaFold, developed by DeepMind (a subsidiary of Alphabet), is making significant strides. This deep learning system has redefined our understanding of protein structures, a cornerstone in the scientific exploration of reverse aging. But how does AlphaFold connect to this quest, and why is its contribution so revolutionary? Let’s delve into the intricacies of this fascinating tool and its potential to transform reverse aging research.
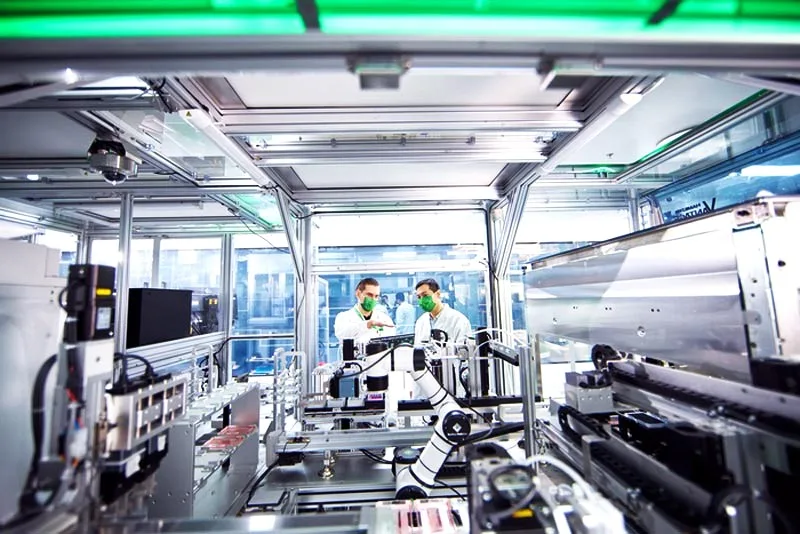
A Leap Forward in Protein Prediction
Proteins, the building blocks of life, dictate virtually every biological process in our bodies. Their functions, ranging from catalyzing biochemical reactions to providing structural support to cells, hinge on their ability to fold into precise three-dimensional shapes. Historically, determining these shapes has been a Herculean task, often requiring years of experimental work. Enter AlphaFold, an AI marvel that predicts protein structures with astonishing accuracy, far beyond traditional methods.
The Backbone of Biological Research: The Protein Data Bank
To appreciate AlphaFold’s innovation, one must understand the Protein Data Bank (PDB), established in 1971. This central archive, maintained by the Worldwide Protein Data Bank (wwPDB), houses all known protein structures determined experimentally. AlphaFold’s ability to accurately predict structures enriches this database, offering insights into previously uncharted biological territories.
AlphaFold: Beyond Protein Folding
AlphaFold’s prowess extends beyond mere protein folding; it excels at predicting structures across ligands, nucleic acids, and post-translational modifications. This broad applicability opens new avenues in drug discovery, exemplified by the AI-driven identification of a novel CDK20 small molecule inhibitor. This discovery marks the first instance of using AlphaFold’s predictions for efficient hit identification, showcasing its potential in streamlining the drug development process.
The Nobel Connection: Christian Anfinsen’s Legacy
The significance of protein structure in biological function was championed by Christian Anfinsen, Ph.D., who was awarded the Nobel Prize in 1972 for his pioneering work. His assertion that a protein’s function is intrinsically linked to its 3D structure underpins the importance of AlphaFold’s contributions. Although exceptions exist, Anfinsen’s principle remains a fundamental tenet in protein science.
AlphaFold and Reverse Aging: A Promising Frontier
The implications of AlphaFold for reverse aging research are profound. Misfolded proteins play a critical role in the pathology of many age-related diseases. By accurately predicting protein structures, AlphaFold enables scientists to understand the mechanisms behind these diseases better and develop targeted therapies. Moreover, its application in drug discovery opens the door to novel treatments that can potentially reverse or mitigate the effects of aging.
Embracing a Future Powered by AlphaFold
AlphaFold represents a paradigm shift in biological research, heralding a new era where AI-driven discoveries accelerate our understanding of life’s fundamental processes. As we continue to harness its potential, the dream of reversing aging becomes increasingly tangible. With each protein structure unraveled, we inch closer to unlocking the secrets of longevity, promising a future where aging as we know it is nothing but a relic of the past.
In conclusion, AlphaFold’s integration into reverse aging research epitomizes the fusion of technology and biology, offering unprecedented opportunities to extend human healthspan. As we stand on the brink of this exciting frontier, one thing is clear: the journey to decode aging has never been more promising.
References:
Nussinov R.; Tsai C. J.; Jang H. Autoinhibition can identify rare driver mutations and advise pharmacology. FASEB J. 2020, 34 (1), 16–29. 10.1096/fj.201901341R. [PubMed] [CrossRef] [Google Scholar]
Park H. M.; Park Y.; Vankerschaver J.; Van Messem A.; De Neve W.; Shim H. Rethinking protein drug design with highly accurate structure prediction of anti-CRISPR proteins. Pharmaceuticals (Basel) 2022, 15 (3), 310. 10.3390/ph15030310. [PMC free article] [PubMed] [CrossRef] [Google Scholar]
Badaczewska-Dawid A. E.; Garcia-Pardo J.; Kuriata A.; Pujols J.; Ventura S.; Kmiecik S. A3D database: Structure-based predictions of protein aggregation for the human proteome. Bioinformatics 2022, 38, 3121. 10.1093/bioinformatics/btac215. [PMC free article] [PubMed] [CrossRef] [Google Scholar]
Song B.; Luo X.; Luo X.; Liu Y.; Niu Z.; Zeng X. Learning spatial structures of proteins improves protein-protein interaction prediction. Brief Bioinform 2022, 23 (2), bbab558. 10.1093/bib/bbab558. [PubMed] [CrossRef] [Google Scholar]
Diwan G. D.; Gonzalez-Sanchez J. C.; Apic G.; Russell R. B. Next generation protein structure predictions and genetic variant interpretation. J. Mol. Biol. 2021, 433 (20), 167180. 10.1016/j.jmb.2021.167180. [PubMed] [CrossRef] [Google Scholar]
Thank you for some other informative site. The place else may just I get that type of information written in such an ideal means? I have a undertaking that I am just now working on, and I have been at the glance out for such information.
Some truly interesting information, well written and broadly speaking user genial.
It’s really a nice and helpful piece of info. I’m glad that you shared this helpful info with us. Please stay us up to date like this. Thank you for sharing.