Advancing Genome Editing with Artificial Intelligence: The Potential of AI-Driven Gene Editors
The frontier of biotechnology is witnessing a significant transformation, thanks to the integration of artificial intelligence (AI) into genome editing. Companies like Profluent are at the forefront, exploring how AI can revolutionize our approach to modifying the human genome. While their AI-generated gene editors are yet to undergo clinical trials, the initial results provide a tantalizing glimpse into the future capabilities of AI in this field.
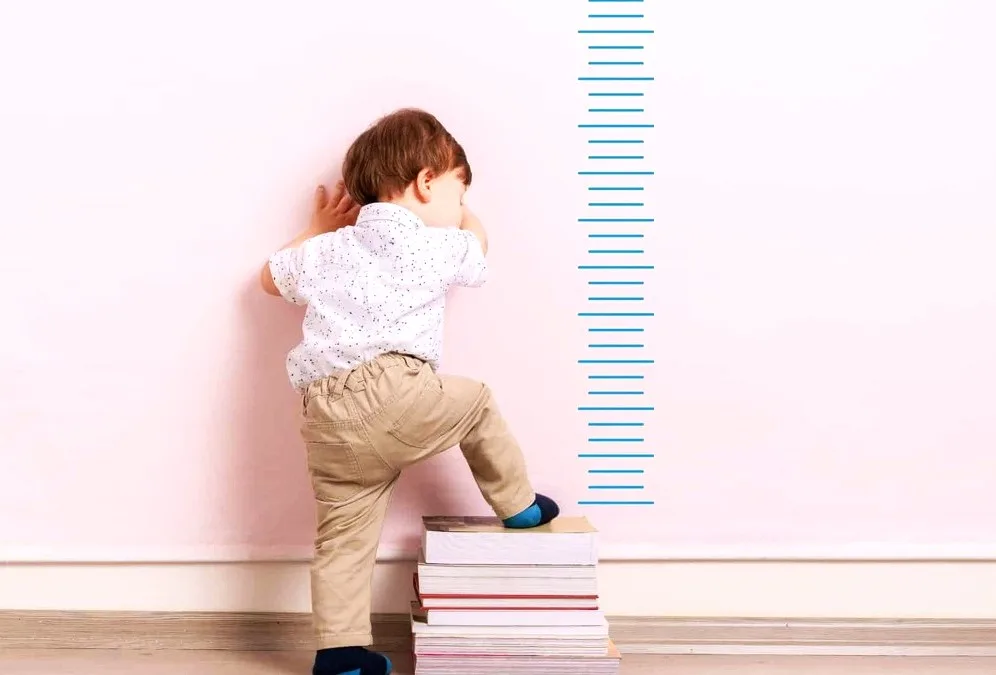
The Role of AI in Genome Editing
AI models, particularly those driven by generative technologies, are becoming a pivotal tool in biotechnology. Generative AI operates on the principles of neural networks—a complex mathematical system that learns from large datasets. For instance, the image generator Midjourney uses a neural network that has studied millions of digital images and their descriptions, learning to associate words with corresponding visuals.
In a similar vein, Profluent’s technology leverages an AI model that comprehends and analyzes sequences of amino acids and nucleic acids. These are the building blocks of the biological processes used to edit genes. By studying the behavior of natural CRISPR gene editors, the AI learns to create entirely new gene editors.
The Promise of Precision and Speed
The most exciting aspect of AI-driven genome editing is its potential for precision and speed. Traditional methods like CRISPR have revolutionized gene editing but come with limitations, including off-target effects and limited editing capabilities for complex genetic conditions. AI has the potential to transcend these limitations by learning from vast amounts of genetic data, enabling the design of gene editors that are both more accurate and versatile.
As AI technologies evolve and ingest more data, their learning accelerates, continuously enhancing their capabilities. Profluent’s ongoing advancements suggest that we might soon have the ability to edit genes with unprecedented precision. The ultimate goal, as highlighted by Dr. Urnov, is to create a future where medical treatments and therapies are rapidly personalized to individual genetic profiles, far surpassing the speed and accuracy of current methods.
Implications and Future Perspectives
While the idea of AI-designed gene editors is promising, it also necessitates cautious optimism. The transition from a proof of concept to clinical application involves rigorous trials and regulatory approvals to ensure safety and efficacy. Furthermore, the ethical implications of such powerful technology cannot be understated. It demands robust ethical frameworks and regulatory guidelines to prevent misuse and ensure that the advancements in genome editing benefit humanity without compromising moral values.
In conclusion, the integration of AI into genome editing opens up a realm of possibilities that could redefine medical science. If technologies like those developed by Profluent continue to advance, we may be on the cusp of a new era in genetics, where diseases are not merely treated but prevented at the most fundamental level. The journey from concept to reality is fraught with challenges, but the potential benefits could reshape our approach to medicine and health care, making personalized treatment a common reality.
References:
Bock C., Datlinger P., Chardon F., Coelho M. A., Dong M. B., Lawson K. A., et al. (2022). High-content CRISPR screening. Nat. Rev. Methods Prim. 2 (1), 8. 10.1038/s43586-021-00093-4 [PMC free article] [PubMed] [CrossRef] [Google Scholar]
Cai L., Fisher A. L., Huang H., Xie Z. (2016). CRISPR-mediated genome editing and human diseases. Genes & Dis. 3 (4), 244–251. 10.1016/j.gendis.2016.07.003 [PMC free article] [PubMed] [CrossRef] [Google Scholar]
Capponi S., Daniels K. G. (2023). Harnessing the power of artificial intelligence to advance cell therapy. Immunol. Rev. 320, 147–165. 10.1111/imr.13236 [PubMed] [CrossRef] [Google Scholar]
Chari R., Mali P., Moosburner M., Church G. M. (2015). Unraveling CRISPR-Cas9 genome engineering parameters via a library-on-library approach. Nat. methods 12 (9), 823–826. 10.1038/nmeth.3473 [PMC free article] [PubMed] [CrossRef] [Google Scholar]
Hamet P., Tremblay J. (2017). Artificial intelligence in medicine. Metabolism 69, S36–S40. 10.1016/j.metabol.2017.01.011 [PubMed] [CrossRef] [Google Scholar]