Revolutionize Drug Discovery with ChemCrow AI
The use of artificial intelligence (AI) in drug discovery is revolutionizing the cutting edge field of pharmaceuticals, greatly increasing the efficacy and efficiency of early-stage research. ChemCrow, an AI system created by researchers at the École Polytechnique Fédérale de Lausanne (EPFL), is a notable innovation in this field. By combining several expert systems, this potent tool can handle challenging chemical research tasks and could completely change the process of finding and creating new medications.
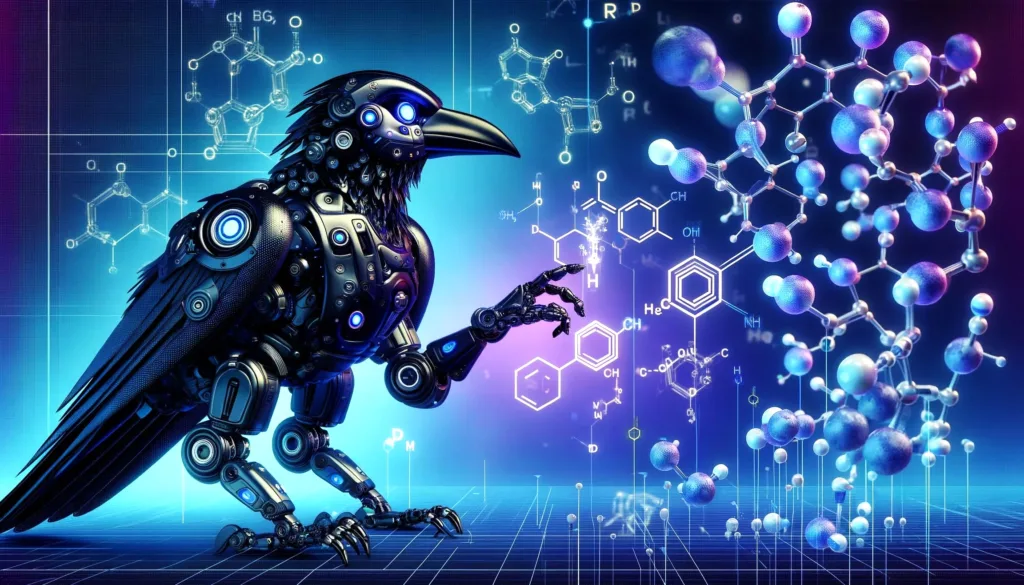
The Importance of Digital Molecular Representation
At the heart of AI-driven drug discovery is the need for precise digital representations of molecules. These digital formats are crucial as they allow machines to process and analyze molecular data efficiently, providing valuable predictions that guide critical decision-making processes in drug development. Over time, molecular representations have evolved significantly:
From Human-Readable to Machine-Readable Formats: Initially, molecular data was formatted in ways that were intuitive for human scientists to read and interpret. However, these were not always ideal for machine processing.
Advancement to Numerical Descriptors: Researchers then moved towards creating bespoke numerical descriptors and fingerprints of molecules, which could be easily handled by computer algorithms.
Adoption of Learned Representations: The latest development involves using AI to learn representations based on patterns and features found in vast chemical datasets. These are particularly effective as they adapt and improve with more data.
Among these, sequence-based and graph-based representations have gained popularity due to their ability to effectively model small molecules, with each method offering unique advantages and presenting certain limitations regarding generality, computational efficiency, reversibility, and interpretability.
ChemCrow: Integrating Expertise for Superior Outcomes
ChemCrow stands out by integrating multiple specialized tools into a single, efficient AI framework. This integration allows ChemCrow to perform a variety of chemical research tasks with remarkable precision and speed. Here’s how ChemCrow is making a difference:
Efficiency in Exploration: By utilizing an integrated approach, ChemCrow can quickly sift through vast chemical spaces to identify promising molecular candidates. This rapid identification significantly cuts down the time and cost typically associated with drug discovery.
Enhanced Predictive Accuracy: ChemCrow leverages advanced AI algorithms to predict molecular properties and biological activities, which are crucial for determining the potential success of new drugs. This predictive capability ensures that only the most promising compounds are moved forward in the drug development pipeline.
Reduction in Failure Rates: Early and accurate predictions help in identifying potential fail points of a compound much earlier in the development process. This not only saves resources but also reduces the overall failure rates in drug discovery programs.
Future Directions and Innovations
As the landscape of drug discovery continues to evolve, AI tools like ChemCrow are at the forefront of innovation. Future developments may include:
Creation of Novel Molecular Representations: There’s ongoing research focused on developing new types of molecular representations, especially for complex, high-value molecules where data is scarce.
Distilling Broader Knowledge: AI systems are increasingly being designed to incorporate wider biological and chemical knowledge, enhancing the depth and accuracy of their predictions.
Modeling New Therapeutic Modalities: With the advent of novel therapeutic approaches, AI tools will need to adapt to model these new modalities effectively.
ChemCrow by EPFL is a prime example of how AI can transform the pharmaceutical industry by making drug discovery faster, cheaper, and more reliable. As AI continues to advance, its integration with expert tools in platforms like ChemCrow is expected to play a pivotal role in shaping the future of medicine, making it an exciting area to watch in the coming years.
By optimizing the digital representation of molecules and integrating advanced predictive models, ChemCrow not only enhances the efficiency of research but also opens new pathways for the development of effective and safe therapeutic solutions.
References:
Bran AM, Cox S. ChemCrow: augmenting large-language models with chemistry tools. In: White AD et al. (eds), 2023. https://arxiv.org/abs/2304.05376.
Schick T, Dwivedi-Yu J, Dessì R, et al. Toolformer: Language Models Can Teach Themselves to Use Tools. 2023. https://arxiv.org/abs/2302.04761.
Boiko DA, MacKnight R, Gomes G. Emergent Autonomous Scientific Research Capabilities of Large Language Models, 2023. https://arxiv.org/abs/2304.05332.
Urbina F, Lentzos F, Invernizzi C, Ekins S. Dual use of artificial-intelligence-powered drug discovery. Nat Mach Intell 2022;4:189–91. [PMC free article] [PubMed] [Google Scholar]
Hay M, Thomas DW, Craighead JL, et al. Clinical development success rates for investigational drugs. Nat Biotechnol 2014;32:40–51. [PubMed] [Google Scholar]
Somebody essentially lend a hand to make significantly posts I might state That is the very first time I frequented your web page and up to now I surprised with the research you made to create this particular put up amazing Excellent job
you are in reality a good webmaster The website loading velocity is amazing It sort of feels that youre doing any distinctive trick Also The contents are masterwork you have done a fantastic job in this topic
you are in reality a good webmaster The website loading velocity is amazing It sort of feels that youre doing any distinctive trick Also The contents are masterwork you have done a fantastic job in this topic